Kubernetes has become the industry standard for orchestrating containerized applications, offering comprehensive tools and methods for scaling, and managing the applications. However, Kubernetes operates as a complex system where multiple processes occur simultaneously due to the dynamic nature of containers and applications. As the scale of application grows, teams often struggle to monitor and keep pace with these dynamic environments, making operations and management increasingly challenging at scale.
To catch up with the pace of events taking place in these dynamic environments, Monitoring is something that can help teams. Monitoring Kubernetes environments allows teams like DevOps and SREs to get real-time information about their containerized applications. Teams can keep track of resources like utilization of memory, CPU, and storage, effective monitoring in Kubernetes helps teams to tackle underlying issues quickly before they become roadblocks. In this blog, we will explore how Prometheus and Grafana help us monitor our Kubernetes environments and see how to set an effective monitoring within Kubernetes.
In this blog, we will understand what Kubernetes monitoring means, and tools that can be leveraged for monitoring our Kubernetes clusters. Also, we will take a look at how Devtron, a Kubernetes management platform helps in integrating monitoring and observability for our Kubernetes application and clusters.
What is Kubernetes Monitoring?
To scale up your applications, provide a reliable service to users, or troubleshoot issues understanding how your application behaves post-deployment becomes crucial. Monitoring acts as the eyes and ears of teams when it comes to keeping track of multiple components of large-scale Kubernetes environments. Setting up monitoring in Kubernetes helps you to continuously examine the containers, pods, services, and Kubernetes clusters. Effective monitoring implementation gives you actionable insights into the problem and underlying issues that enable teams to quickly perform troubleshooting as they know where the problem is happening or are about to happen.
What is Prometheus?
Prometheus is an open-source monitoring and alerting toolkit developed by SoundCloud and later donated to the Cloud Native Computing Foundation (CNCF). Prometheus excels in collecting and storing the metrics from its targets, which can be applications, servers, databases, or any component of the system that exposes the metrics through an HTTP endpoint. The metrics data fetched by Prometheus is stored in its time series database which can be queried using Prometheus Query Language (PromQL).
Prometheus is made up of multiple different components that allow Prometheus to effectively monitor and trigger alerts on its target.
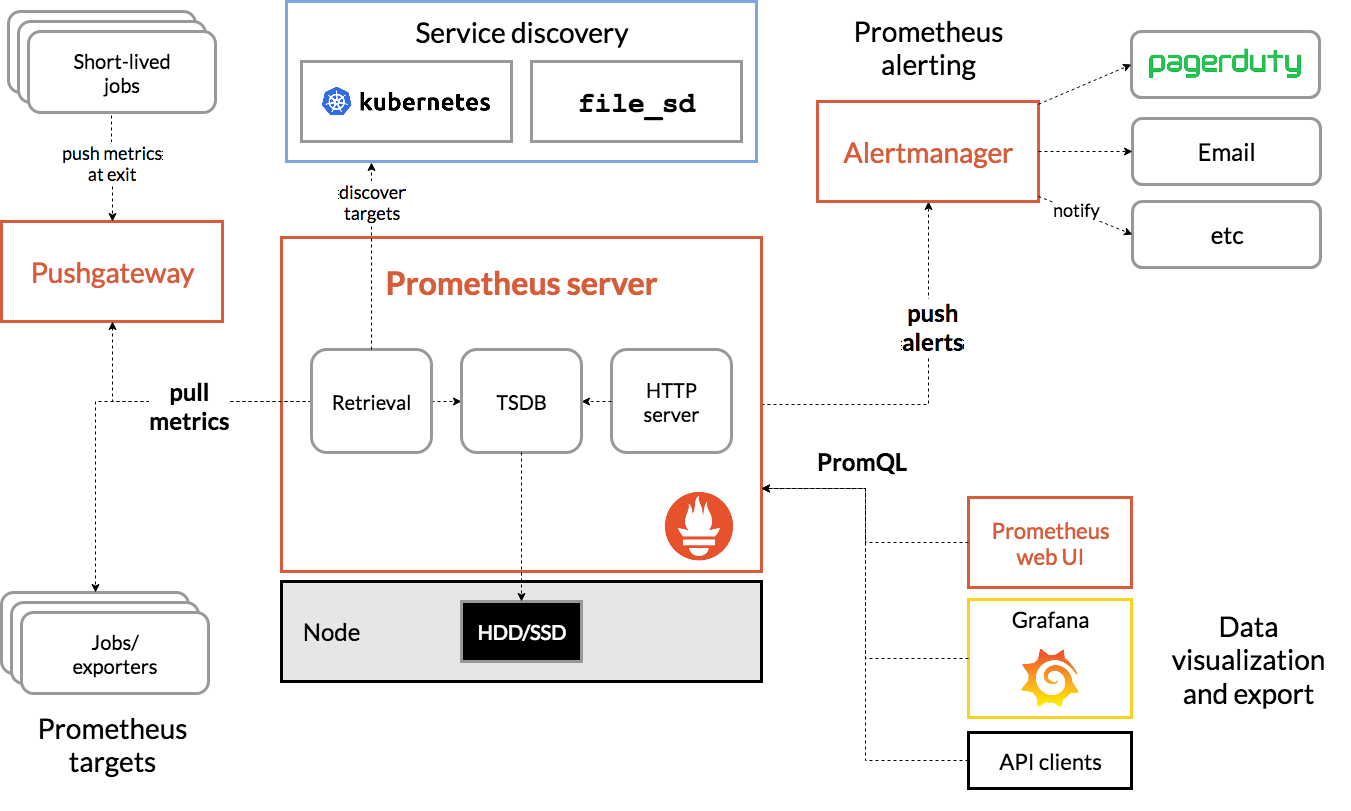
The key components of Prometheus are:
Prometheus Server
Prometheus Server is the core component of Prometheus that handles metric collection, storage, and serving of time-series data. It pulls metrics from configured targets at specified intervals, stores them locally or remotely, and provides a powerful query language (PromQL) for data analysis. Think of it as the central brain that manages all your monitoring data.
Prometheus Alertmanager
Prometheus Alertmanager manages alerts sent by the Prometheus server. It handles alert grouping, deduplication, silencing, and routing notifications to various services like email, Slack, or PagerDuty. When specified alert conditions are met, Alertmanager ensures the right people are notified through their preferred channels.
Prometheus Targets
Prometheus Targets represent the endpoints that Prometheus monitors. These could be your applications, services, or infrastructure components that expose metrics at an HTTP endpoint (usually /metrics). Each target provides metrics in the Prometheus format that the server scrapes at regular intervals.
Pushgateway
Pushgateway is a component that allows you to push metrics to Prometheus, rather than having them pulled. It's particularly useful for short-lived jobs or batch processes that may not exist long enough for Prometheus to scrape them. Think of it as a metrics buffer that accepts pushed data and holds it until Prometheus pulls it.
Service Discovery
Service Discovery helps Prometheus automatically find and monitor new targets in dynamic environments like Kubernetes. Instead of manually configuring each target, service discovery can automatically detect new services, pods, or instances and update Prometheus's scrape configurations accordingly. This is crucial in cloud-native environments where services come and go frequently.
Prometheus Exporter
Prometheus Exporters are specialized components that convert metrics from existing systems (that don't natively expose Prometheus metrics) into a format Prometheus can understand. For example, the Node Exporter converts system metrics (CPU, memory, disk usage) into Prometheus metrics, while the MySQL Exporter does the same for MySQL database metrics. Exporters act as bridges between your existing systems and Prometheus.
What is Grafana?
Grafana is an open-source interactive and data-visualization platform developed by Grafana Labs. Grafana helps users to query, visualize, explore metrics, and monitor data no matter where they are stored with the help of insightful and customized dashboards and visualizations. You can connect Grafana dashboards with any data sources like Prometheus, MySQL, Influx DB, ElasticSearch, PostgreSQL, etc. Some key features of Grafana are:
Custom Dashboards
The Grafana dashboard is a set of one or multiple panels, organized into a single pane of glass which provides an at-a-glance view of all key metrics. These panels are customizable and users can create them using components that query and transform raw metrics data from data sources into charts, graphs, and other visualizations.
Alerts
Grafa allows users to set up alerts that notify them of the specific conditions or anomalies in their data i.e. in logs and metrics.
Data Source Compatibility
Grafana supports a wide variety of data sources users can store their raw metrics data in time series databases like Prometheus and CloudWatch, logging tools like Loki and Elasticsearch, NoSQL/SQL databases like Postgres, CI/CD tooling like GithHub, and many more.
Kubernetes Monitoring with Prometheus and Grafana
In the dynamic environments of Kubernetes, monitoring Kubernetes clusters has become crucial for maintaining robust and reliable applications. Prometheus and Grafana form a powerful combination that has emerged as the de facto standard for Kubernetes monitoring. While Prometheus excels at collecting and storing time-series metrics from your Kubernetes clusters, pods, and containers, Grafana transforms this raw data into insightful visualizations and dashboards. Together, they provide DevOps teams with real-time visibility into cluster health, resource utilization, application performance, and potential bottlenecks. This monitoring stack not only helps in proactive issue detection but also enables data-driven decision-making for scaling and optimization.
When it comes to deployment of these tools into Kubernetes environments, users can use Kube-Prometheus-Stack, The stack automatically configures service discovery for your Kubernetes components and sets up default monitoring rules. It includes predefined alerts, recording rules, and basic Grafana dashboards.
Now, let’s take a look at how you can deploy monitoring stacks into your Kubernetes environments using Devtron.
Monitoring Stack with Devtron
Devtron has been built in a modular fashion where you can install different integrations as per your requirements. For monitoring, the default integration is Grafana, as mentioned above. Once Grafana has been installed, now we need to set up a metrics collector, which would scrape all the metrics and act as a data source for Grafana. In this tutorial, we will set up Prometheus and add it to the Devtron dashboard for fetching application metrics.
Grafana
To use the Grafana dashboard, you need to first install the integration from the Devtron Stack Manager. The beauty of integrations that Devtron brings in is that users don't have to worry about the different tools and their complexities. All the heavy lifting is carried out by Devtron and users just need to interact with the Devtron dashboard. In case, you want to access the Grafana dashboard, you need to port forward the devtron-grafana
service if Devtron is running in the local system or exposes it via ingress.
kubectl -n devtroncd port-forward svc/devtron-grafana 3000:80 &
Go to your browser and type the URL, In our case, it is localhost:3000, and you should see this. Click on the sign-in button at the bottom left corner.
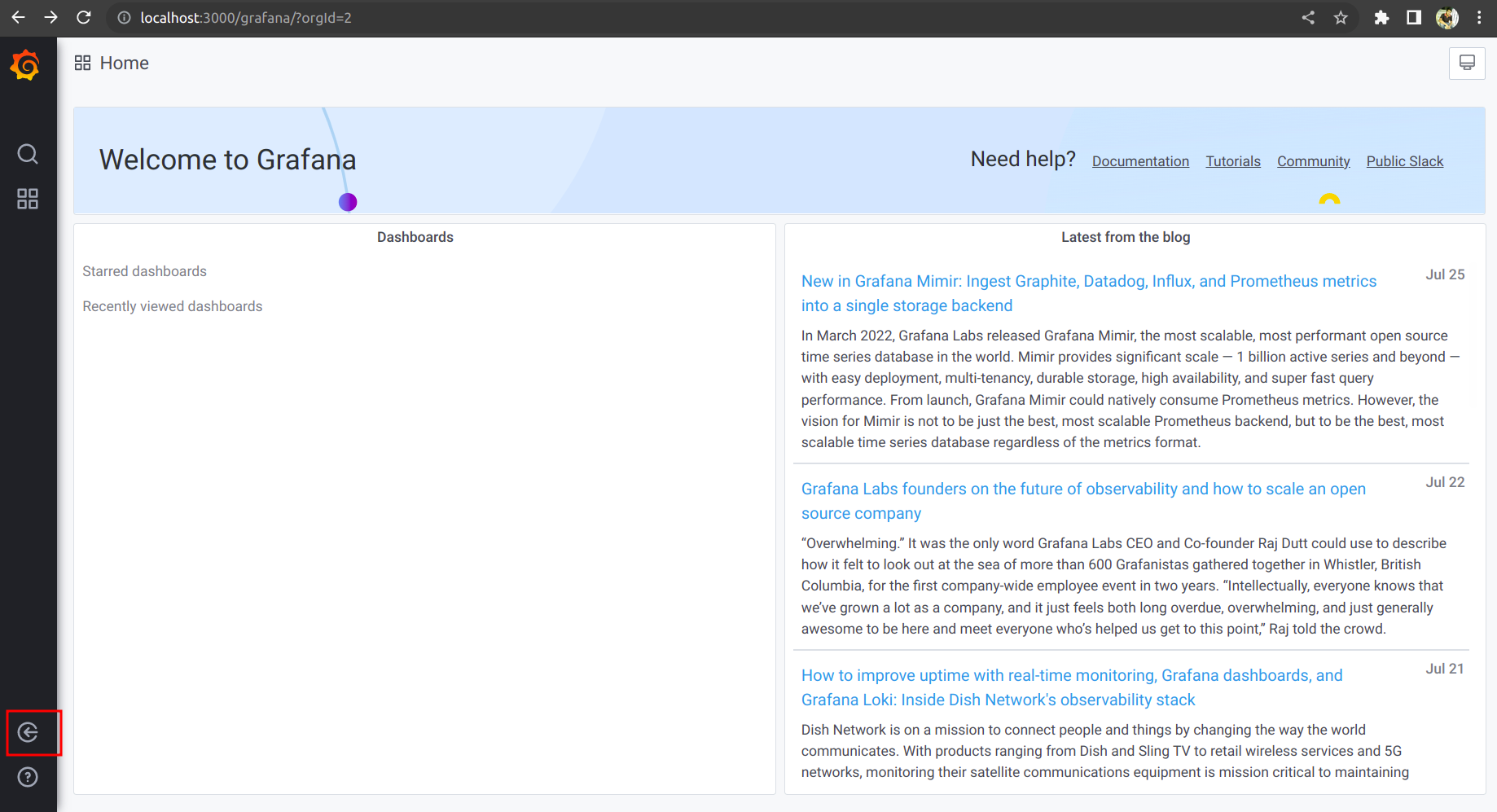
You should see this on the login page of Grafana.
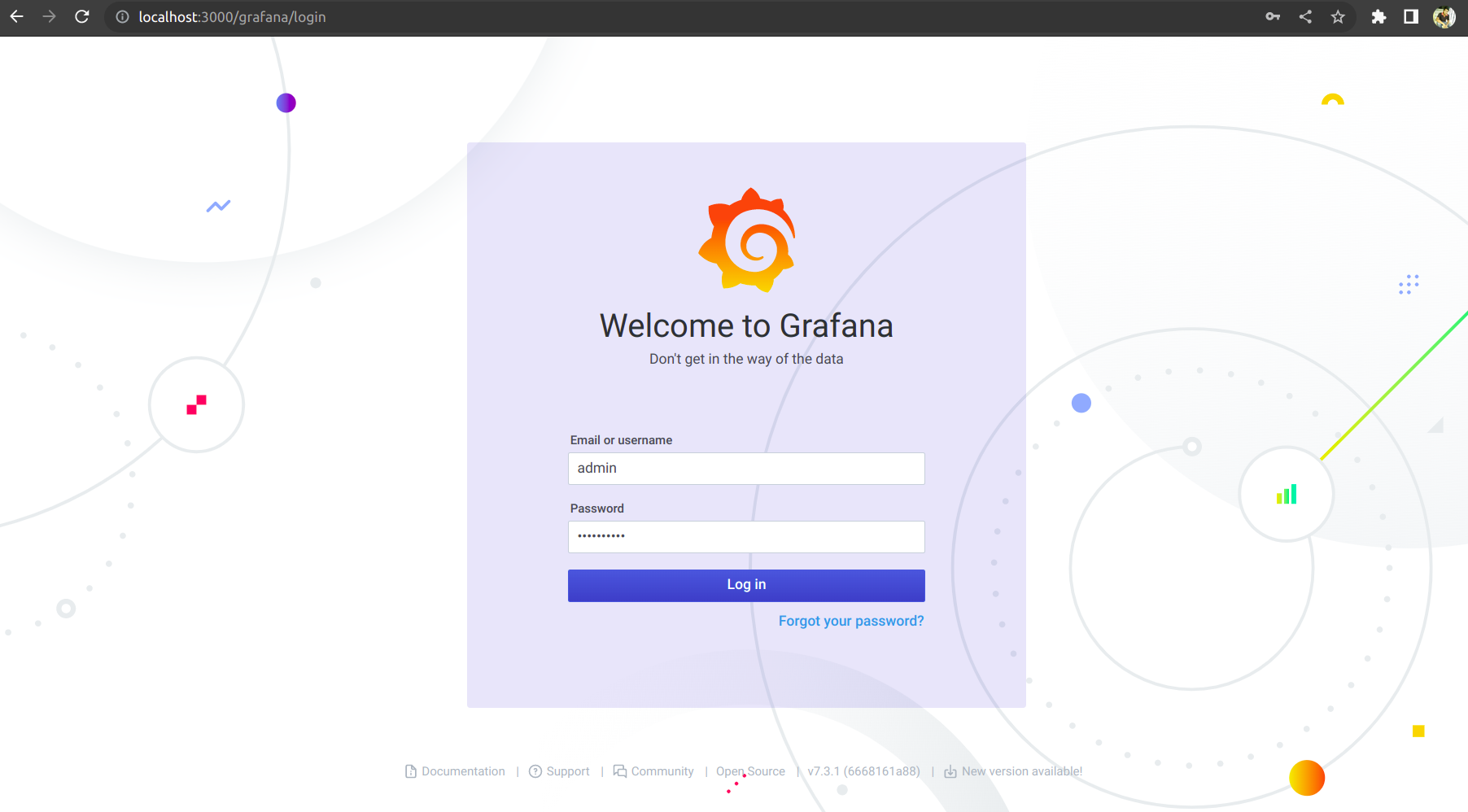
To login into the Grafana dashboard, your username is admin
and you can get your password by executing the following command:
kubectl get secrets -ndevtroncd devtron-grafana-cred-secret -ojsonpath='{.data.admin-password}'|base64 -d
Installing the Prometheus required CRDs
Before we can go ahead and install Prometheus from the chart store, we first need to install the Custom Resource Definitions that are needed for running Prometheus. These CRDs do not get applied by the helm chart, hence we have to do it manually.
The manifest files that we will require for applying CRDs exist within the kube-prometheus GitHub repository. There are multiple manifest files that have to be applied. So let’s first go ahead and clone the repository. Run the below command to clone the repository to your local system.
git clone https://github.com/prometheus-operator/kube-prometheus && cd kube-prometheus
The manifests that we require exist within the manifests/setup
directory. However, simply applying these manifests will throw an error. These manifests have to be applied on the server side. We can do this by passing the --server-side
flag within the command. Run the below command to apply the CRDs.
kubectl apply --server-side -f manifests/setup
Alternatively, we can apply the CRDs directly from the raw files which exist within the same repository. If you wish to do that, run the below command:
kubectl apply --server-side -f https://raw.githubusercontent.com/prometheus-operator/kube-prometheus/main/manifests/setup/0alertmanagerConfigCustomResourceDefinition.yaml
kubectl apply --server-side -f https://raw.githubusercontent.com/prometheus-operator/kube-prometheus/main/manifests/setup/0alertmanagerCustomResourceDefinition.yaml
kubectl apply --server-side -f https://raw.githubusercontent.com/prometheus-operator/kube-prometheus/main/manifests/setup/0podmonitorCustomResourceDefinition.yaml
kubectl apply --server-side -f https://raw.githubusercontent.com/prometheus-operator/kube-prometheus/main/manifests/setup/0probeCustomResourceDefinition.yaml
kubectl apply --server-side -f https://raw.githubusercontent.com/prometheus-operator/kube-prometheus/main/manifests/setup/0prometheusCustomResourceDefinition.yaml
kubectl apply --server-side -f https://raw.githubusercontent.com/prometheus-operator/kube-prometheus/main/manifests/setup/0prometheusagentCustomResourceDefinition.yaml
kubectl apply --server-side -f https://raw.githubusercontent.com/prometheus-operator/kube-prometheus/main/manifests/setup/0prometheusruleCustomResourceDefinition.yaml
kubectl apply --server-side -f https://raw.githubusercontent.com/prometheus-operator/kube-prometheus/main/manifests/setup/0prometheusruleCustomResourceDefinition.yaml
kubectl apply --server-side -f https://raw.githubusercontent.com/prometheus-operator/kube-prometheus/main/manifests/setup/0scrapeconfigCustomResourceDefinition.yaml
kubectl apply --server-side -f https://raw.githubusercontent.com/prometheus-operator/kube-prometheus/main/manifests/setup/0servicemonitorCustomResourceDefinition.yaml
kubectl apply --server-side -f https://raw.githubusercontent.com/prometheus-operator/kube-prometheus/main/manifests/setup/0thanosrulerCustomResourceDefinition.yaml
kubectl apply --server-side -f https://raw.githubusercontent.com/prometheus-operator/kube-prometheus/main/manifests/setup/namespace.yaml
Now that we have the CRDs applied within the cluster, we can go ahead and install Prometheus
Note: Please make sure that you apply the CRDs before installing the kube-promethus stack. Otherwise, certain components may not be created.
Installing Prometheus
Go to the chart store and search for Prometheus. Use the Prometheus community's kube-prometheus-stack
chart to deploy Prometheus. To learn more about this chart, check out the official chart page.
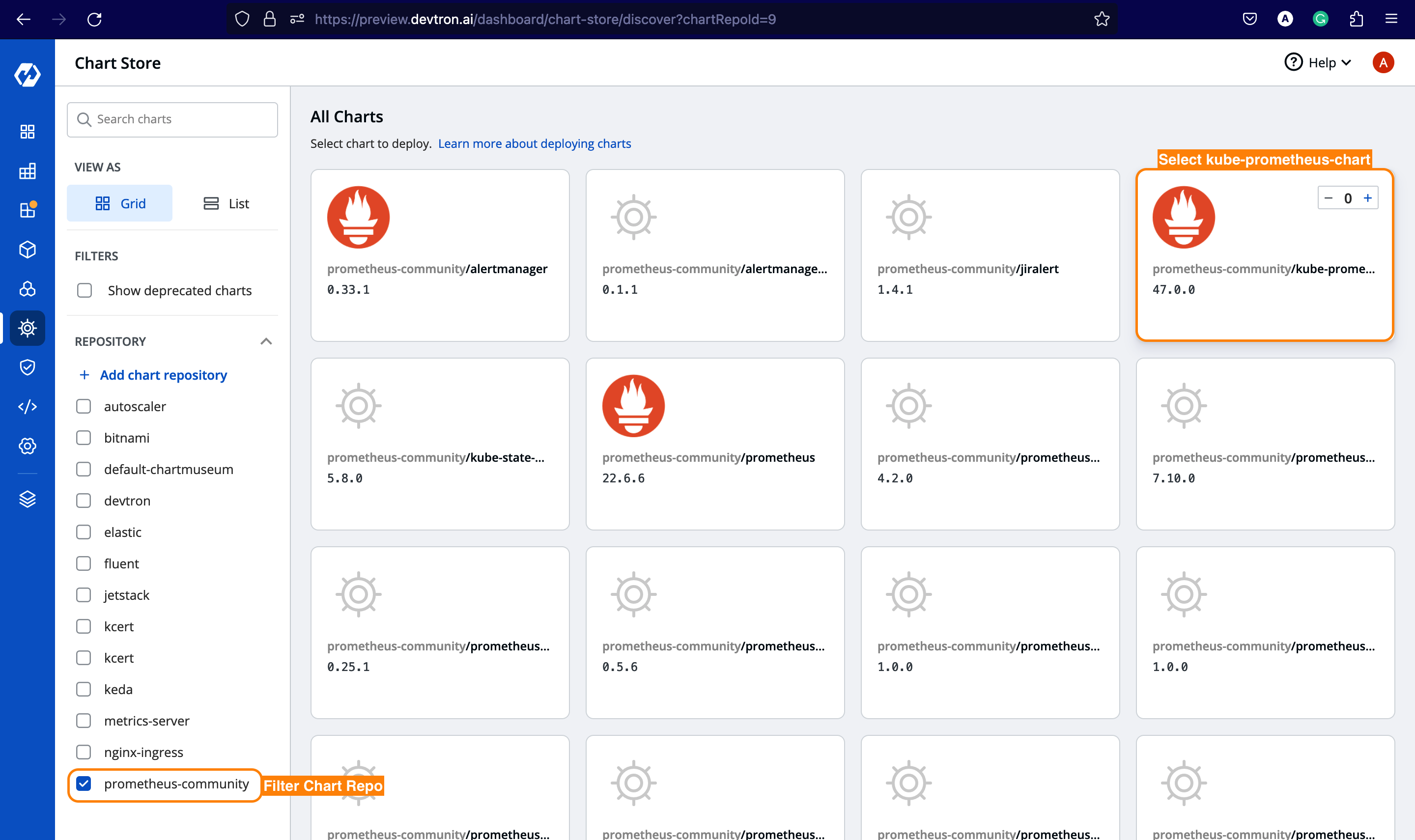
Once you select a chart, you must configure the values per your requirements before deploying. In our case, let's make the following changes.
kube-state-metrics:
metricLabelsAllowlist:
- pods=[*]
serviceMonitorSelectorNilUsesHelmValues: false
podMonitorSelectorNilUsesHelmValues: false
Search for the above parameters and set these values as defined above. Additionally, if you want to expose the Prometheus dashboard via ingress, you can enable the ingress and provide the hostname for it, and likewise for Grafana which is being bundled with kube-prometheus-chart
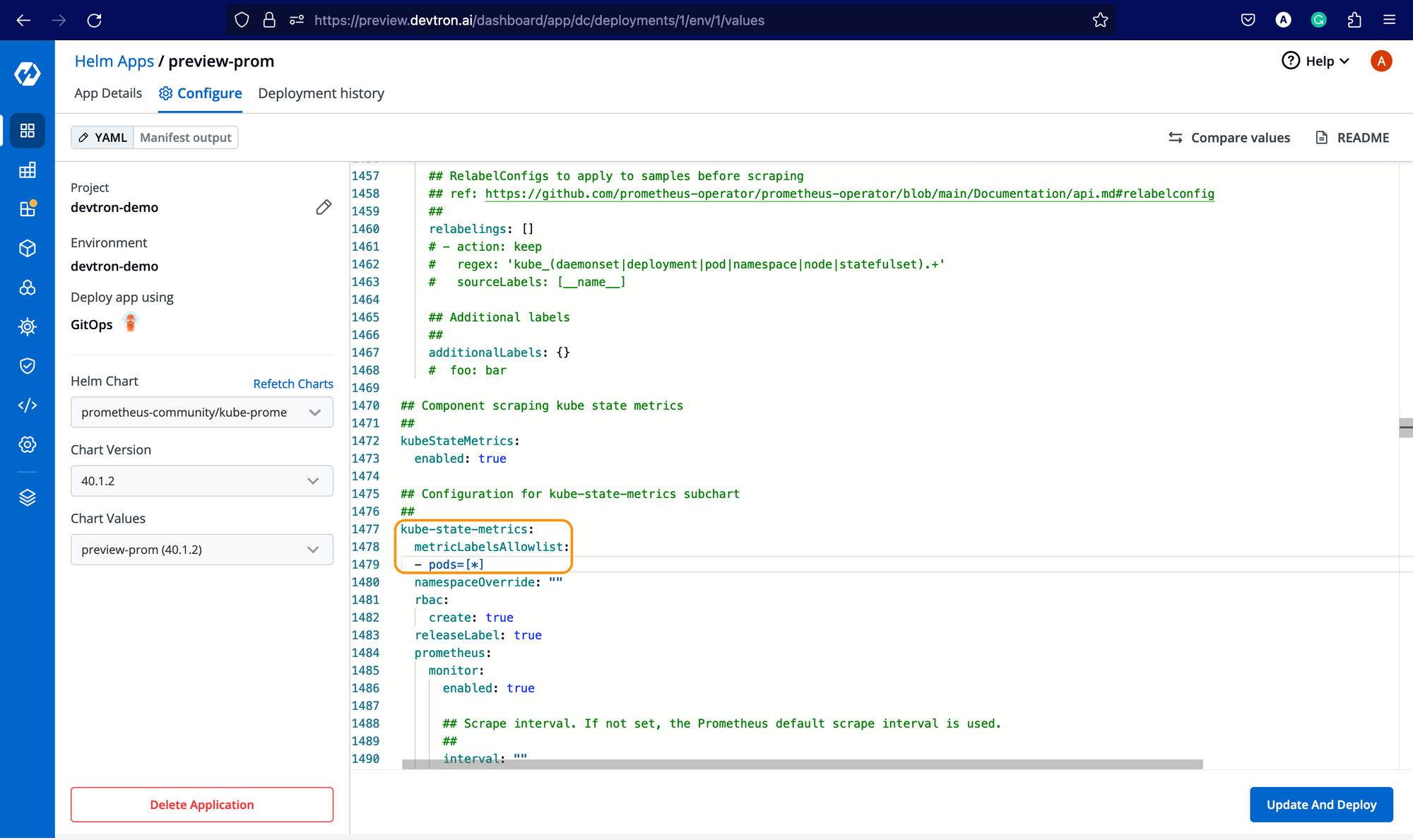
Now, after installing Prometheus, you need to get the endpoint of the Prometheus server. For every helm chart or application deployed at Devtron, you get a resource-grouped view for all Kubernetes resources deployed along with him. To get the endpoint, under Networking
go-to service
expand the Prometheus server service and you should be able to see the Endpoints along with EndpointSlice as shown below.
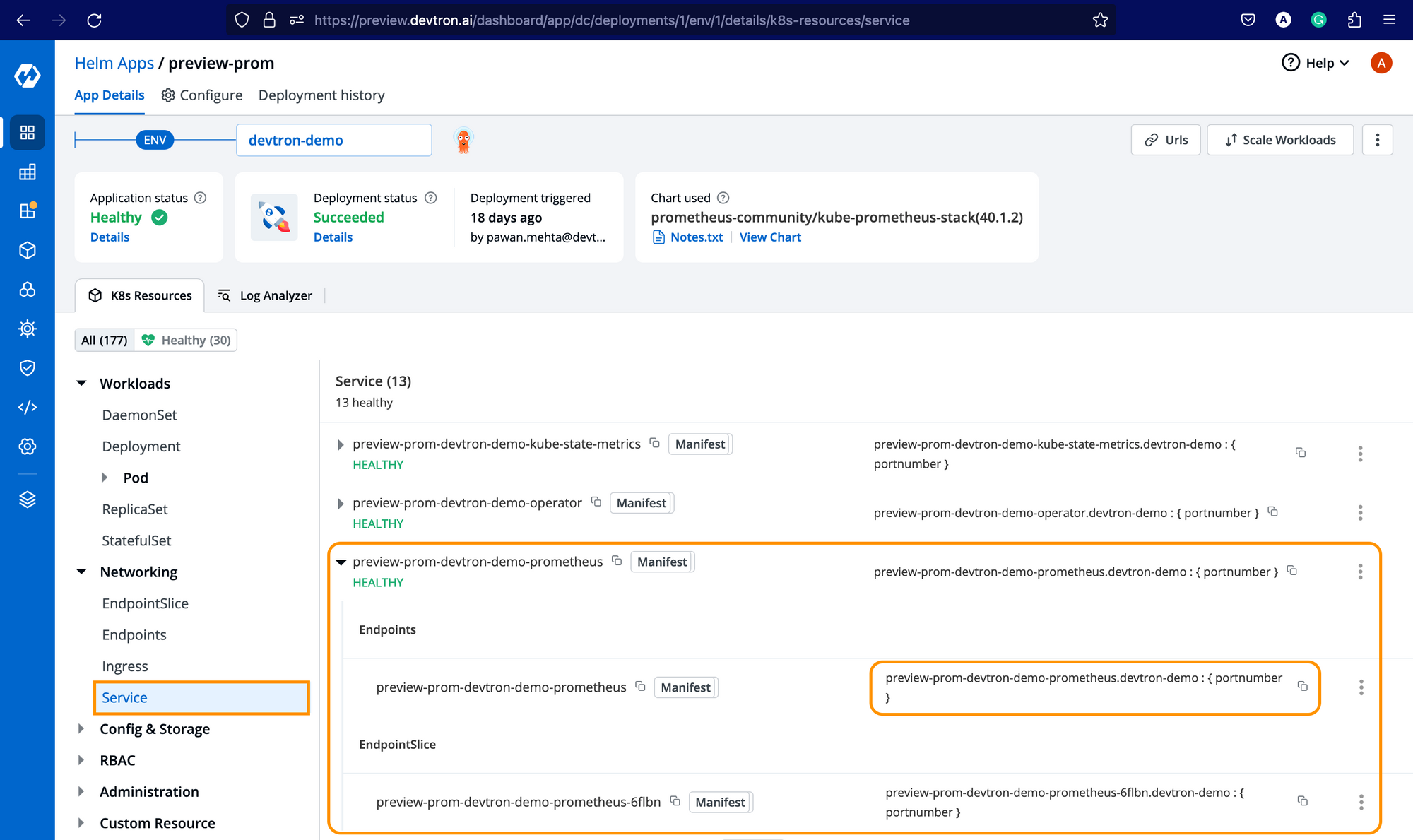
Enable Application Metrics
Not to use Prometheus as a data source for Grafana, go to Global Configurations
-> Clusters & Environments
and for the respective cluster where you have installed the Prometheus chart, add the endpoint as mentioned below and click on Update Cluster
.
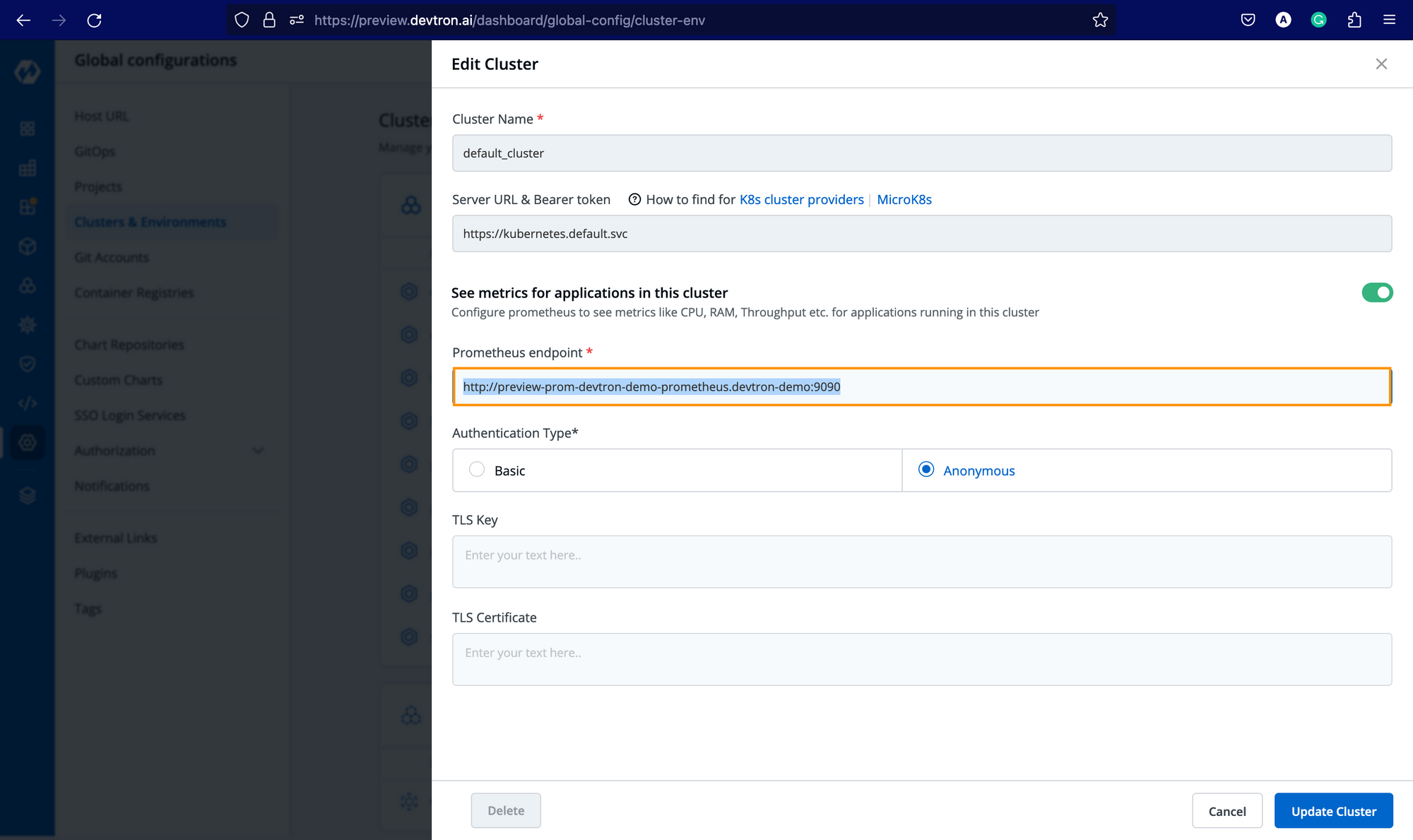
Once you add the endpoint, you will be able to see the application metrics in the Devtron dashboard for all the applications deployed in the respective cluster irrespective of the environment (ns) it is being deployed.
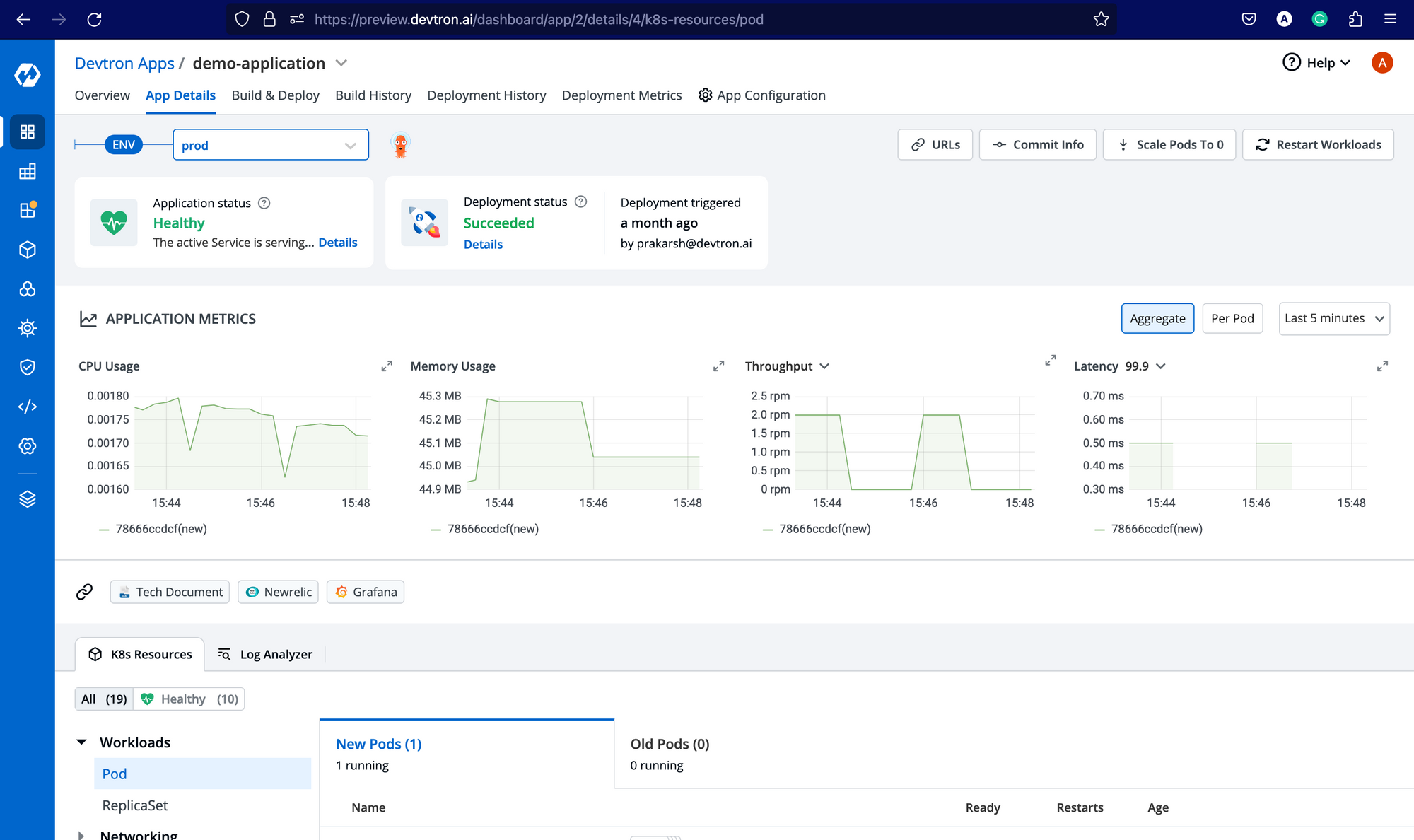
With this, you will be able to track your application metrics like resource usage, CPU usage, Throughput, and Latency. For Throughput and Latency, you need to enable the application metrics for respective environments from Deployment Template
as mentioned below.
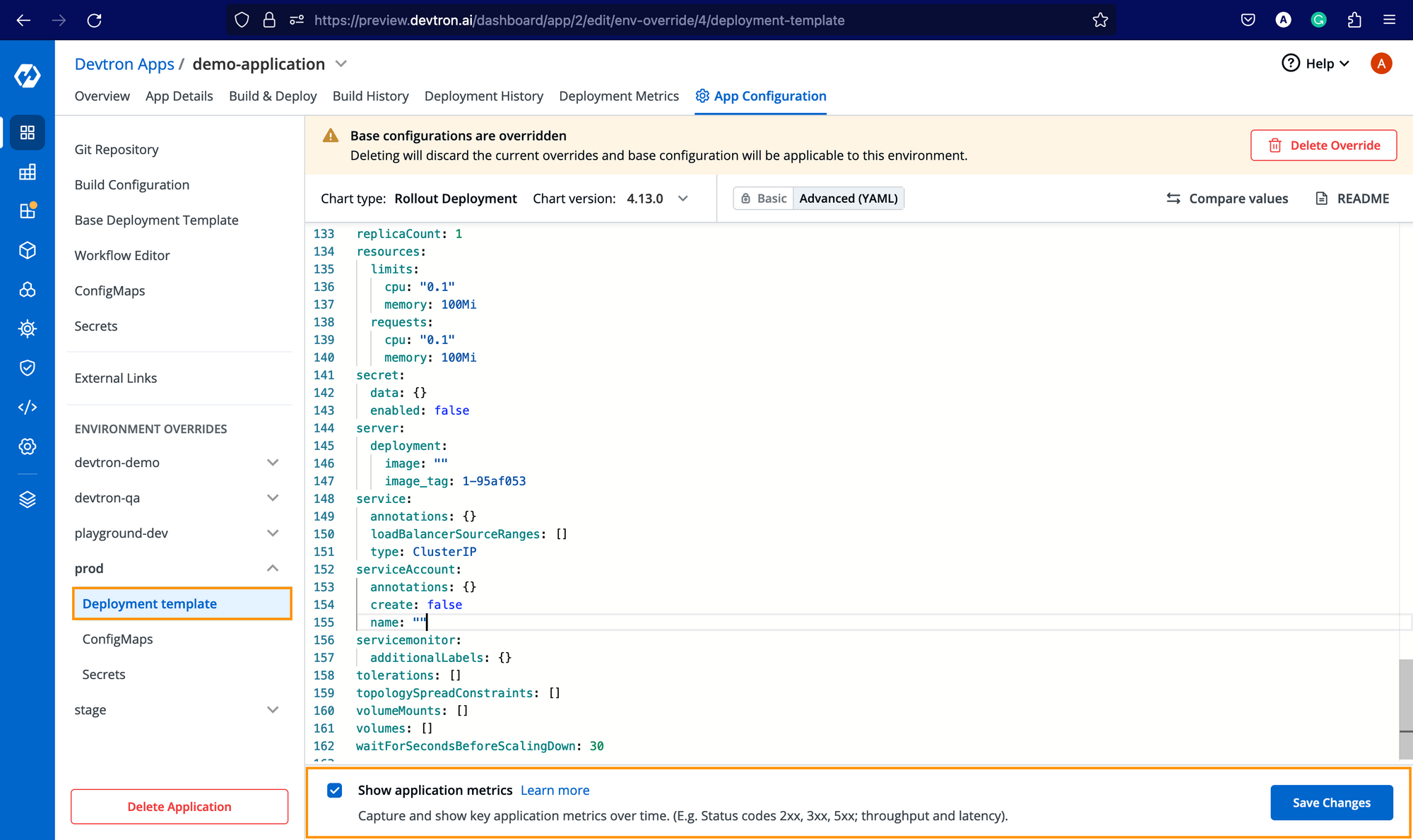
Conclusion
This is how you can set up a monitoring stack for Kubernetes with Devtron and easily observe and monitor all your applications. You can also use other tools such as Robusta, Pixie, New-relic, and other monitoring tools with Devtron, as it provides the flexibility to integrate with any tool of your choice.
Feel free to check out the Devtron documentation for more info, and if you like Devtron, do give it a star on GitHub.